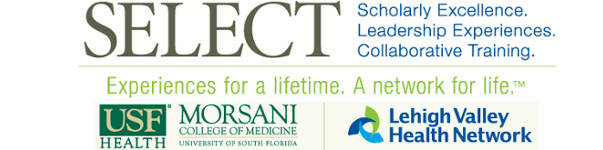
USF-LVHN SELECT
Recipient Survival after Orthotopic Liver Transplantation: Interpretable Machine Learning Survival Tree Algorithm for Patient Specific Outcomes.
Publication/Presentation Date
1-5-2023
Abstract
BACKGROUND: Elucidating contributors affecting liver transplant survival is paramount. Current methods offer crude global group outcomes. To refine patient-specific mortality probability estimation and determine covariate interaction using recipient and donor data, we generated a survival tree algorithm (ReSOLT) using UNOS transplant data.
STUDY DESIGN: The UNOS database was queried for liver transplants in patients ≥18 years old between 2000-2021. Pre-operative factors were evaluated with stepwise logistic regression; 43 significant factors were used in survival tree modeling. Graft survivalcalculated.
RESULTS: A total of 122,134 liver transplant patients were included for modeling. Multivariable logistic regression (AUC = 0.742, F1 = 0.822) and survival tree modeling returned 8 significant recipient survival factors: recipient age, donor age, recipient primary payment, recipient Hepatitis C status, recipient diabetes, recipient functional status at registration and at transplantation, and deceased donor pulmonary infection. Twenty subgroups consisting of combinations of these factors were identified with distinct Kaplan-Meier survival curves (p
CONCLUSIONS: Survival trees are a flexible and effective approach to understand the effects and interactions of covariates on survival. Individualized survival probability following liver transplant is possible with ReSOLT, allowing for more coherent patient and family counseling and prediction of patient outcome using both recipient and donor factors.
ISSN
1879-1190
Published In/Presented At
Rogers, M. P., Janjua, H. M., Read, M., Cios, K., Kundu, M. G., Pietrobon, R., & Kuo, P. C. (2023). Recipient Survival after Orthotopic Liver Transplantation: Interpretable Machine Learning Survival Tree Algorithm for Patient Specific Outcomes. Journal of the American College of Surgeons, 10.1097/XCS.0000000000000545. Advance online publication. https://doi.org/10.1097/XCS.0000000000000545
Disciplines
Medical Education | Medicine and Health Sciences
PubMedID
36728472
Department(s)
USF-LVHN SELECT Program, USF-LVHN SELECT Program Students
Document Type
Article