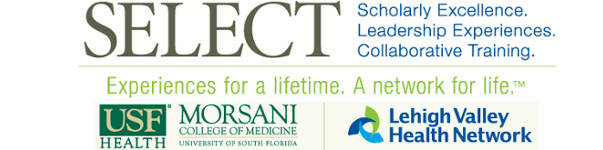
USF-LVHN SELECT
Evaluating clinical and radiomic features for predicting lung cancer recurrence pre- and post-tumor resection.
Publication/Presentation Date
2-1-2024
Abstract
Among patients with early-stage non-small cell lung cancer (NSCLC) undergoing surgical resection, identifying who is at high-risk of recurrence can inform clinical guidelines with respect to more aggressive follow-up and/or adjuvant therapy. While predicting recurrence based on pre-surgical resection data is ideal, clinically important pathological features are only evaluated postoperatively. Therefore, we developed two supervised classification models to assess the importance of pre- and post-surgical features for predicting 5-year recurrence. An integrated dataset was generated by combining clinical covariates and radiomic features calculated from pre-surgical computed tomography images. After removing correlated radiomic features, the SHapley Additive exPlanations (SHAP) method was used to measure feature importance and select relevant features. Binary classification was performed using a Support Vector Machine, followed by a feature ablation study assessing the impact of radiomic and clinical features. We demonstrate that the post-surgical model significantly outperforms the pre-surgical model in predicting lung cancer recurrence, with tumor pathological features and peritumoral radiomic features contributing significantly to the model's performance.
Volume
12926
ISSN
0277-786X
Published In/Presented At
Ho, W. L. J., Fetisov, N., Hall, L. O., Goldgof, D., & Schabath, M. B. (2024). Evaluating clinical and radiomic features for predicting lung cancer recurrence pre- and post-tumor resection. Proceedings of SPIE--the International Society for Optical Engineering, 12926, 1292623. https://doi.org/10.1117/12.3006091
Disciplines
Medical Education | Medicine and Health Sciences
PubMedID
38993353
Department(s)
USF-LVHN SELECT Program, USF-LVHN SELECT Program Students
Document Type
Article